AI Automation in Large Enterprises: A Journey Through Hidden Complexity
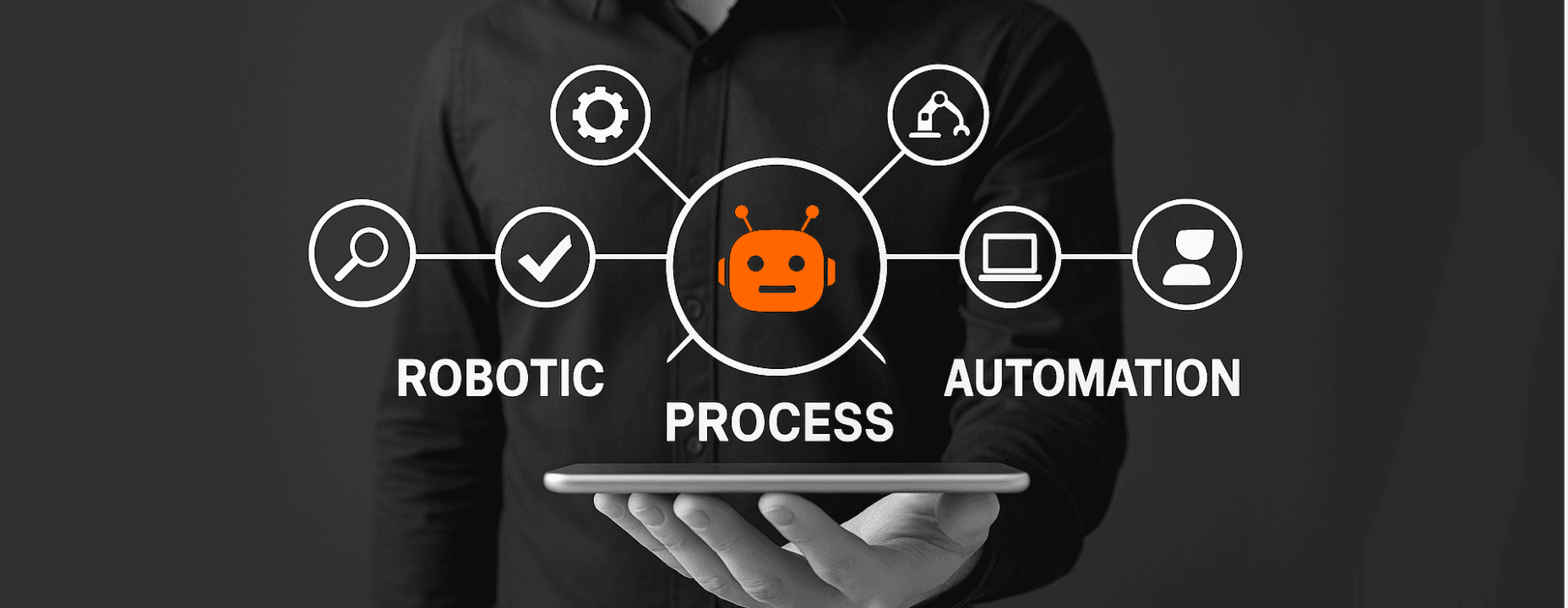
The popular image of AI automation in enterprises often borders on fiction: diligent bots executing repetitive tasks, streamlined workflows with just a few clicks, and sleek dashboards signaling the future. However, when the stage is the large-scale corporate environment, automation is no longer just a technical matter, it becomes a structural shift.
In this context, automating with AI is not merely about saving time or improving operational efficiency. It is, above all, an exercise in confronting unresolved processes, outdated structures, and decisions that, out of convenience or fear, have gone unquestioned for years.
This article is based on a clear premise: true AI automation in the enterprise world is not a smooth upgrade. It is a rupture. And like any rupture, it demands preparation, courage, and a deep understanding of the terrain.
The Misleading Promise of “The Bot That Does Everything”
In the corporate imagination, automation often comes wrapped in reassuring promises.
RPA tools, generic copilots, and automated assistants are sold as plug-and-play solutions, tools that operate on top of existing realities without interfering with the organizational fabric.
The metaphor of the “bot that creates reports on its own” is seductive: it reinforces the notion that automation is incremental, not disruptive; that it is technical, not political; that it solves problems without stirring discomfort.
But this fantasy doesn’t survive contact with the real world of large corporations.
There, processes are anything but linear. They are layered with exceptions, workarounds, and historic decisions that were never revisited.
Legacy systems are not just outdated, they’re deeply embedded. And the operational flows that support day-to-day routines often function in spite of their structure, not because of it.
The Problem Is Not Technical, It’s Structural
In large-scale AI automation initiatives, the biggest obstacle is rarely technological. Tools are available. So is technical talent. The blockage lies elsewhere: in politics, operations, and often, emotions.
Every automatable process is surrounded by power dynamics, comfort zones, and unspoken agreements. There are process owners who don’t want to give up control.
There are silos protected by corporate cultures resistant to change. There are exceptions that became the rule, not because they were efficient, but because it was easier to work around them than to fix them.
In this environment, automation means much more than implementing technology. It means reopening buried discussions, questioning flows that have survived through inertia, and challenging structures that were designed to remain untouched.
Automation as a Tool for Organizational Reconstruction
This is where intelligent AI-powered automation finds its true value, not as a productivity tool, but as a catalyst for organizational transformation.
By tackling the institutional complexity that sustains existing processes, well-executed automation projects force a revision of operational foundations. And this is not a side effect, it’s a strategic opportunity.
When well planned, these projects become true Trojan horses: they enter through the door of efficiency and, once inside, expose silos, illuminate bottlenecks, reveal the dysfunctional logic behind many routines, and force long-avoided decisions.
In this scenario, automation isn’t about layering AI on top of chaos. It’s about reorganizing the chaos. It’s about turning exceptions into clear rules. Creating interoperability where there was noise. Rebuilding the operational flow based on logic, data, and intentionality, not on legacy, power, or fear.
Process Engineering Comes Before AI
No AI, no matter how advanced, can fix a poorly designed business process on its own. Before the predictive model, the generative assistant, or the latest RPA system, you need process engineering.
That means deeply mapping each step, understanding its true (and not always declared) objectives, identifying interdependencies, bottlenecks, and friction points. It requires listening to the silence between systems. Noticing the data that doesn’t flow. The approvals that drag on. The exceptions that pile up.
Only with this level of contextual understanding is it possible to apply AI effectively and sustainably.
Conclusion
AI automation in large enterprises is not a technology project, it’s an organizational maturity project. Those who approach it with depth, embracing discomfort, breaking through silos, and redesigning processes with courage, gain real advantages: predictability, scalability, and operational margin.
Those who treat automation as cosmetic, layering solutions on top of fragile structures, merely accelerate existing problems. With a prettier interface, perhaps, but the same old dysfunctions.
The future of intelligent automation doesn’t belong to those looking for shortcuts. It belongs to those willing to open the black box, revisit what no one else wants to touch, and build, with AI, a new operational logic: clearer, faster, and above all, more honest.